Adobe PDF
(2.79 MB)
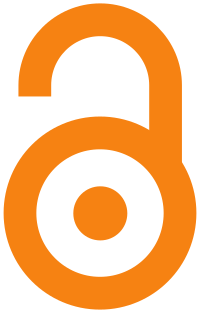
Seiten Aufrufe
355
aufgerufen am 19.11.2023
Download(s)
109
aufgerufen am 19.11.2023
