Adobe PDF
(1.74 MB)
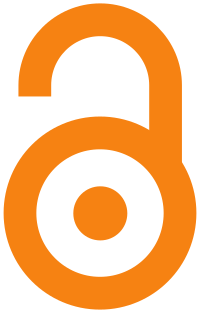
Page view(s)
191
checked on Jan 23, 2025
Download(s)
78
checked on Jan 23, 2025
