Adobe PDF
(4.93 MB)
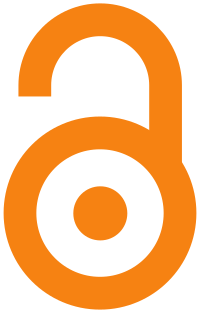
Page view(s)
452
checked on Jun 18, 2024
Download(s)
200
checked on Jun 18, 2024
