Adobe PDF
(8.52 MB)
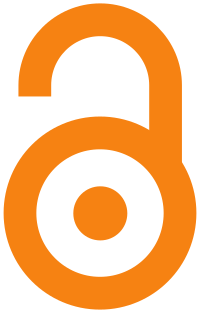
Seiten Aufrufe
88
aufgerufen am 05.12.2024
Download(s)
126
aufgerufen am 05.12.2024
