Adobe PDF
(2.43 MB)
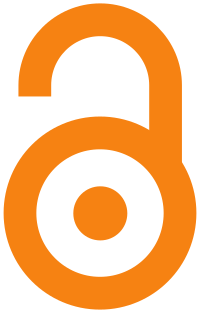
Seiten Aufrufe
486
aufgerufen am 19.11.2023
Download(s)
228
aufgerufen am 19.11.2023
